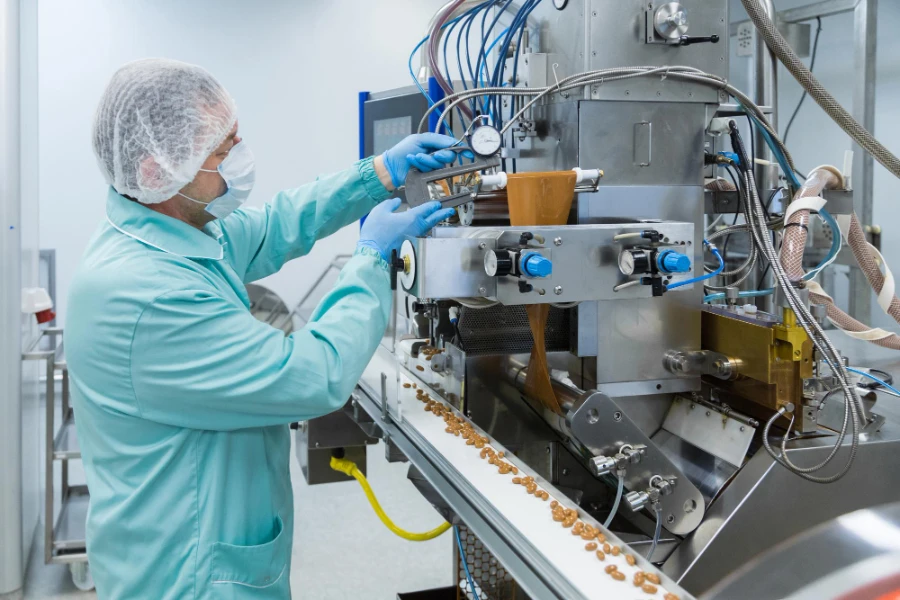
Artificial intelligence and machine learning are powering major breakthroughs across many industries. However, in research dependent industries like pharmaceuticals, these technologies are progressing faster. AI is mining insights from multiple data sets making it highly interoperable and reshaping the value chain to bring life-saving drugs to us faster.
Will it impact every stage of the pharma value chain, from molecule to market? Let’s explore.
Drug discovery
Drug discovery is often a manual, labour & capital intensive process, one that can be augmented through the use of AI. Biopharma companies can explore AI models to identify & validate several processes and use the findings to improve their predictive capabilities.
In molecular design, generative modeling for molecule design & protein engineering could be well-impacted with AI. Where clinical trials require manual data inputs, AI driven data flows can integrate trial data from numerous sources and create standardized digital data that down streams to relevant systems on its own.
Manufacturing
Avoiding laborious manual intervention, AI in biopharma manufacturing could stream vital data, predict bottlenecks in processes, assess quality control issues at hand and suggest appropriate corrections. Allowing a tighter control, it could reduce manual oversight in manufacturing operations and operating costs with:
- Quality control
- AI in robotic process automation (RPA)
- AI simulations to optimize yield & output
- AI-enabled predictive maintenance to reduce machine downtime
Supply chain
To better prepare for supply chain disruptions, AI could automate demand planning, supply chain and marketing data to predict demand & supply, provide recommendations while autonomously performing certain activities.
Moving away from conventional supply chains, dynamic digital supply networks (DSNs) could be used to leverage technologies like sensors and advanced analytics that streamline production & distribution.
Commercial & Marketing
Consumer engagement in biopharma tends to address larger groups rather than precise targeted consumers based on preferences. In such cases, AI engagement solutions for omni channels take into consideration “how”, “when” and “what” type of communication will engage patients as well as health care practitioners (HCPs).
Beyond the scope of marketing, AI can be used to analyze & resolve patient grievances, queries and social media data to transform products & services. Conversational AI can be used to increase patient literacy while providing them with better treatment options.
Assembling the Building Blocks
- Whether an ERP system or other technologies, the aim to implement AI must be to work cohesively with existing technological systems, so organizations can invest in designing & developing the right data standards.
- Up scaling AI requires a considerable investment in the right IT architecture & infrastructure. Begin with identifying which technological, data, and systems capabilities are required to upscale the use of AI.
- A talent strategy to understand skill availability within the organization will make transitioning to AI smoother in comparison to the scarcity of data scientist talent.
- Aligning AI-risk management with the organization’s risk approach will need to be incorporated along with training of AI teams to safeguarding intellectual property.
On a wider scale, AI must be transparent & understandable, robust & reliable. CLOUDSUFI ensures a transparent & ethical use of AI across the organization with a full suite of capabilities and assets with a full service spectrum across the life sciences value chain, including technologies for data, analytics, intelligent automation, and machine learning.