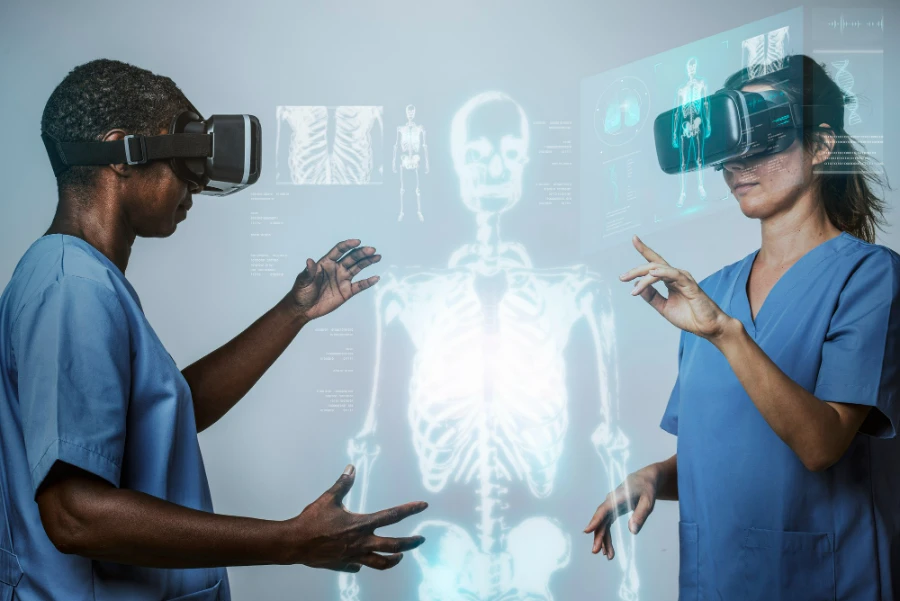
As technology advances, AI, specifically the subset of AI – machine learning (ML), is an essential part of an increasing number of medical devices. Deep learning techniques to diagnose cancer, deter anomalies in CT scans, robotics in surgery, and neural networks to determine ideal drug candidates for testing are just a few examples healthcare is witnessing.
AI-based devices though routinely used to monitor patient health & journey, AI-based healthcare products have now begun to rephrase our understanding of the potential AI holds.
Trends in Medical Devices with AI
Although the scope of AI exceeds far beyond what it is today, medical device companies are developing devices to serve many functions, a few among which are:
- Chronic Disease Management – By closely monitoring patient health, such devices could deliver treatment without the guidance of a doctor. In diabetic patients, AI can administer insulin dosage as per the patient’s blood sugar levels.
- Medical Imaging – To reduce a patient’s radiation exposure, companies incorporate AI-driven technology in machines that improve accurate clinical results.
- Telehealth – Combining chatbot technologies with AI systems can help patients self-diagnose themselves. Powered by Natural Language Processing, chatbots though not ready to provide primary diagnosis, can assist medical professionals by equipping them with the information they can obtain.
From a Regulatory Point of View
Today, there are not many guidelines that explicitly control the use of AI in medical devices. The U.S. Food & Drug Administration attempts to clarify its usage through 8 guiding principles. Laying the foundation of “Good Machine Learning Practice”, it outlines points that international standards organizations and other collaborative bodies can follow.
The principles of the first pillar are:
- The total product life cycle considers multidisciplinary expertise.
- Sound software engineering and security practices are used for model design.
- The aimed patient population is represented in the participant & data sets.
- The best available methods are chosen to select reference data sets.
- Model design is modified as per available data and describes intended device use.
- The performance of the human-AI team is considered primary.
- Device performance during actual clinically relevant events is prioritized.
- Models deployed that have a precise modus operandi are be monitored and performance risks are well managed.
Following a Total Product Lifecycle (TPLC), the FDA advocates the following:
1. Safety and effectiveness review prior to market launch
2. Establishing pre-specifications and algorithm change protocol
3. Patient transparency and performance monitoring in real-world
Future of Tech
While some of the biggest players in the healthcare sector and employing AI/ML to map healthcare data, deliver brilliant solutions and better patient outcomes, strong regulation & close monitoring of post-market safety must be maintained by users of this technology.
For sure, AI has earned a spot in medical device development in-spite of a few roadblocks and lapses. With these removed & the development framework strengthened, biomedical engineers and healthcare professionals can create novel medical technologies. This will reduce incidences of wrongful diagnosis among a few and pave the way for innovative patient-friendly functionalities.